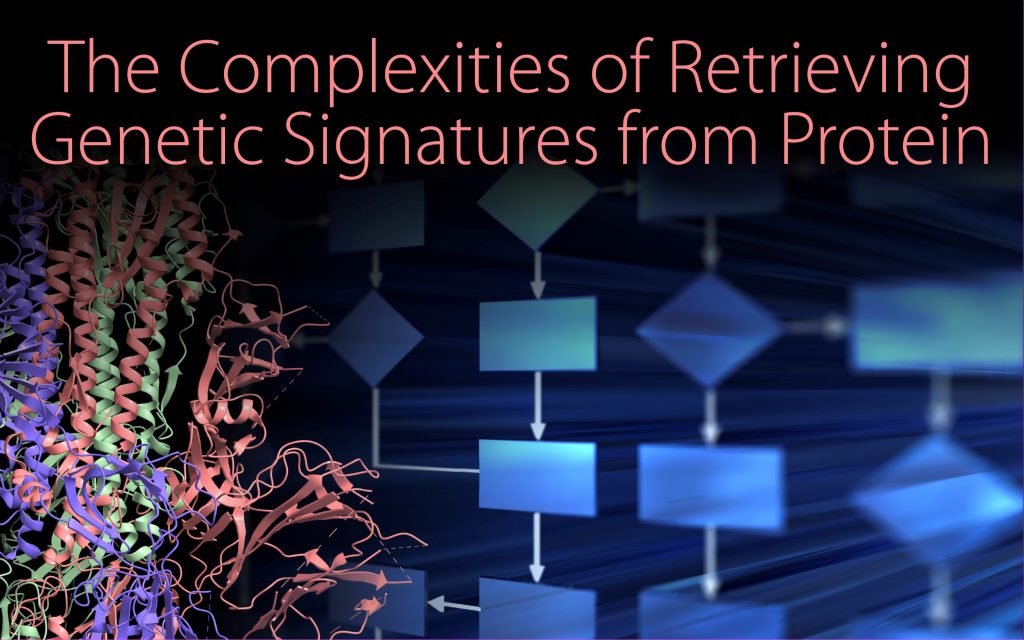
Abstract
Forensic genetic investigations typically rely on analysis of DNA for attribution purposes. There are times, however, when the amount and/or the quality of the DNA is limited, and thus little or no information can be obtained regarding the source of the sample. An alternative biochemical target that also contains genetic signatures is protein. One class of genetic signatures is protein polymorphisms that are a direct consequence of simple/single/short nucleotide polymorphisms (SNPs) in DNA. However, to interpret protein polymorphisms in a forensic context, certain complexities must be understood and addressed. These complexities include: 1) SNPs can generate 0, 1, or arbitrarily many polymorphisms in a polypeptide; and 2) as an object of expression that is modulated by alleles, genes and interactions with the environment, proteins may be present or absent in a given sample. To address these issues, a novel approach was taken to generate the expected protein alleles in a reference sample based on whole genome (or exome) sequence data and assess the significance of the evidence using a haplotype-based semi-continuous likelihood algorithm that leverages whole proteome data. Converting the genomic information into the proteomic information allows for the zero-to-many relationship between SNPs and GVPs to be abstracted away. When viewed as a haplotype, many GVPs that correspond to the same SNP is equivalent to many SNPs in perfect linkage disequilibrium (LD). As long as the likelihood formulation correctly accounts for LD, the correspondence between the SNP and the proteome can be safely neglected. Tests were performed on simulated samples, including single-source and two-person mixtures, and the power of using a classical semi-continuous likelihood versus one that has been adapted to neglect drop-out was compared. Additionally, summary statistics and a rudimentary set of decision guidelines were introduced to help identify mixtures from protein data.
Read full paper here.
Keywords
Genetically variable peptides, Massively parallel sequencing, Proteomics, Probabilistic genotyping, Mixtures.
Authors
August E.Woernera,b, Benjamin Crysupa, F. CurtisHewittc, Myles W. Gardnerc, Michael A.Freitasd,e, Bruce Budowlea,b
a Center for Human Identification, University of North Texas Health Science Center, Fort Worth, TX, USA
b Department of Microbiology, Immunology and Genetics, University of North Texas Health Science Center, Fort Worth, TX, USA
c Signature Science, LLC, Austin, TX, USA
dThe Ohio State University, Columbus, OH, USA
eThe Ohio State University Wexner Medical Center, Columbus, OH, USA